Is there a better way to set up the RPI?
By Jeff Taylor
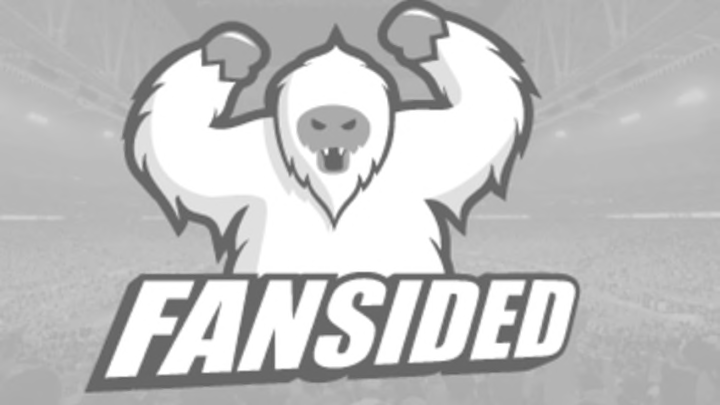
This is part II of the series looking at the usefulness of the Ratings Percentage Index in comparing and ranking college basketball teams and whether it can be improved to give better guidance for NCAA committee members in selecting and seeding teams for the NCAA tournament.
As I mentioned in Part I, the RPI does not take into account WHO you beat or by how many points. It simply looks at your winning percentage and that of your opponents. But, when I ran the two scenarios with UW being 3-1, many of you thought the one with UW beating Duke on the road, but losing to Arkansas was more impressive than the one where UW lost to Duke, but beat Arkansas. However, when you calculated both scenarios, the RPI value remained the same at 0.5375
So, what if the RPI were tweaked to take that into account?
In a scenario I created, each team’s RPI becomes a multiplier value which you can place onto the games to give extra value to quality wins and lower values to wins over lesser opponents. Say for instance Duke is the #1 team in the RPI out of 347 teams. Then, you could make their multiplier worth 1.347. Houston Baptist is the #347 team in the RPI, so their multiplier is 1.001. Each position a team is in the RPI is worth 0.001 more than the one below them. Beyond that, the RPI formula is the same.
When you run the numbers you see that UW’s new RPI for WP in scenario #1 is 1.021, while their new RPI for WP in scenario #2 is 0.735. Compare those totals to the original WP value of 0.650. In this situation, the multiplier value really gives an added boost to the fact that UW beat the #1 team, as opposed to the #110 team. It does not give any penalties for what might be considered a bad loss to Arkansas though. But, the loss is still taken into account by the Win-Loss record. This multiplier can be seen as a way to see the highest potential a team can reach.
If you wanted to also take into account whether a game was won on a last second buzzer beater or in a blowout, you could also assign multipliers based on scoring differential. This is used by many of the alternate rankings systems out there already. However, there are some inherent problems with such multipliers. Here are a few:
1) When a team is desperate late in the game and intentionally fouling, the other team can quickly turn a 3 point lead to 10 by shooting free throws.
2) Once a team is up by 20+ in a game, they may start playing reserves that will allow the score to be much closer at the end than it otherwise would have been.
3) In reality, the difference between a 15 point win and a 30 point win is just noise based on the effort teams are putting in on both sides late and does not really show the quality differential between teams.
So, you have to ask yourself, do you want coaches substituting based on perceived bonus points or loss of points? Should Romar be afraid to play Sherrer because their 21 point lead might drop to 18 before the end of the game? Do you want teams who are down 6 with 20 seconds left to stop trying so that they can preserve a 6 point loss, rather than risk it going up to 10 with intentional fouls? And, do you really want teams up 28 points late in the game to put in all their starters so they can get it up to 30 points before the buzzer?
So, if you are going to give bonus points for scoring differential, it either needs to be so minimal as to not affect the decision making of the coaches, or make the intervals so far apart as to not encourage changes in substitution patterns (maybe only at 10 points and 20 points?).
There are other methods out there for ranking teams of course. For instance, Ken Pomeroy has the Pomeroy College Ratings, which is not used to rank teams per say, but rather as a prediction of future outcomes. His ratings are based on Pythagorean expectations. While not getting into the nitty-gritty statistical analysis of how that works (But, I do have graduate level statistics courses on my transcripts!), it basically compares the number of points a team scores to the number of points a team gives up. As you might imagine, if a team scores more than it gives up, it tends to win a lot of games.
– It should be noted that Pomeroy uses an exponent of 11.5
However, Pomeroy adjusts those numbers above by taking into account points per 100 possessions as offensive efficiency, as well as, the defensive efficiency of the opponent since some teams (Tony Bennett come to mind?) simply will not allow high scoring teams to score as much as they normally would, even in a loss). There is also the “luck” factor, which in essence looks at the deviation from the expectations for every team.
Analysis shows that Pomeroy’s index is 2% more predictive than the RPI over time.
Another analysis used is the Sagarin Ratings. Sagarin does not publish his methology, but it appears he uses a variation of the Elo rating system used in Chess, which in essence takes into account both the win/loss record plus the ranking of the competitors (similar to the method I used above). Sagarin also applies a point differential to his ratings for predictive purposes. Sagarin’s method is considered the best by gamblers in setting point spreads.
There is one other factor that must be considered; the referees. Statistical analysis by Dean Oliver showed that even neutral unbiased referees making “random” errors end up favoring the underdog over time. In fact, the underdog has a 5% greater chance of winning a game if referees screw up 15% of their calls/no calls. We all know that 15% error rate is not beyond the realm of possibilities for Pac-12 referees!
In addition, some referees call games tighter than others. If some referees call quick fouls on aggressive defensive teams, they can quickly get players in foul trouble, change the minutes played and dynamics of the game, resulting in different results. The reverse can also hold true allowing teams to get away with too much. So, there really is some luck of the draw in who is officiating on the court and how that may affect the final outcome.
Whether any of these methods really work “best” for ranking teams is up for argument. The old “any given day” adage still holds true. It is very difficult to account for a myriad of factors such as injuries and illness, travel wearyness, the effects of school work (mid-terms and finals), team discipline issues and the emotional state of the players, one player having a career day, and the random nature of referees, that true predictions and rankings to a fine degree are almost impossible. With only 5 players on the floor for each team and 3 referees, the effect just one of them can have on any given day is enormous.
However, over the course of an entire 30-33 game season, the data eventually begins to even out and many of the outliers start to have less of an effect on the final result. In reality, these ranking systems work better in basketball than in football since there simply is much more data to work with. While some of the methods out there may be slightly better than the RPI, in the end the RPI ends up giving a pretty good sense for how teams rank compared to each other.
I still think a system that rewards teams for victories against stronger opponents, rather than just simply win/loss totals, gives a better distinction to the highest abilities a team can reach. But, until the NCAA changes its system, teams need to schedule using the current RPI as their metric.
In part III of the series, I’ll examine whether Lorenzo Romar schedules UW to maximize the RPI potential for the NCAA tournament.